The below is an excerpt from the foreword of the 2020 Sharp Football Preview book.
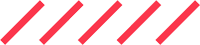
Since the invention of football, the sport has been supported by numbers. As far back as those numbers go, there has been a quest to find better ones. Things we’re exploring now aren’t exactly new ideas.
In Dr. Z’s A Thinking Man’s Guide To Pro Football, there’s a chapter on statistics. In it, he talks about the folly of counting total tackles, which can come at all depths of the field and can vary in frequency given the home stadium scorer. He also talked about how passer rating is a jumbled mess that no one understands. That book was first published in 1970 with an updated version released in 1984.
Then there was The Hidden Game of Football, published in 1988, which also touched on the limits of traditional NFL statistics and started to introduce things like success rate, which helped shape some modern analysis.
Fast forwarding, Football Outsiders has been writing about why the number of rushing attempts in a game is not the reason for a team winning since the site’s first article in 2003. Rather, the best indicator is having a lead late in games, although you wouldn’t know it based on the fact that there’s still a debate on this topic today.
In 2020, there is more data and better information than we’ve ever had before. There are so many advanced stats based on play-by-play and now there are even metrics based on player tracking from Next Gen Stats. All of this information can feel overwhelming, like the nerds decided to create a secret language just for themselves. But the good news is you really don’t have to be all that deep into math to figure out that so many of these ideas just make sense once you take some time to understand them. I know from experience.
I was a Communications major in college and needed no more than two math classes to graduate. So like a good Communications major, I sought out and satisfied the minimum requirement. After taking a freshman-level math class during my first semester, I took a class called “Digital Mapping,” which counted as my second math in the second semester of my freshman year. It was quite literally a class about Google Maps and how similar programs worked. There was little actual math involved. My athletics academic advisor at the time was even impressed by my ability to find that class and avoid any real math altogether. That was the last official math class I ever took.
All of that is to say that despite my aforementioned extensive math background, I have been able to get a handle on these concepts and statistics and now use them regularly.
What Is EPA?
Why don’t we kick things off with Expected Points Added (EPA)? One of the common counters to analytics is how it lacks context, but EPA packs just about as much context as possible into one number. Every play has a value and because football has been around for so long, we are able to find historical values of similar plays based on the spot, down and distance, time, and score.
What EPA essentially does is give each situation on the field a value based on what has historically been done at that same spot. For example, we can see what a typical offense has done in the past on a 1st and 10 at its own 40-yard line. Using that as a baseline, we can see how much better or worse a current play was from that same spot on the field.
By using yards, there’s no difference between an 8-yard gain on 2nd and 7 or an 8-yard gain on 3rd and 14. The raw difference there is the basis of success rate, one of the first forays into a more advanced football stat, but EPA takes that a few steps further. There’s also a difference between a four-yard gain on 3rd and 3 and a 12-yard gain on 3rd and 15. All of this gets accounted for in EPA. The first play obviously helps an offense more despite the second gaining three times as many yards.
Of course, EPA isn’t perfect. Despite being based on historical play-by-play, there is not one universal baseline for expected points. That can cause some slight differences based on where specific EPA data comes from, but that’s not a massive drawback and nowhere near a good enough reason to discount the value it brings to the table.
Ideally, EPA can be paired with success rate — or rate of plays that produce positive EPA — to give a full look at value and consistency as opposed to a few big plays bumping up total EPA.
Because EPA is based on historical play-by-play and offenses have evolved, the average EPA over a season is not zero. In 2019, the average pass was worth 0.02 EPA and the average run was worth -0.05. That brings us to…
What We’ve Learned About the Run Game
One of the biggest impacts of analytics over the past decade-plus has been what it revealed about the run game. What we know is that throwing the ball is more efficient than running. The above EPA numbers reflect that, but the advantage is clear even if you take the average yards gained on such plays. In 2019, the average yards per attempt on a pass was 7.2 while the average run gained 4.3 yards. That’s a slight oversimplification, but it helps shape how we’ve thought about the run game and its impact incorrectly for too long.
The early 2000’s saw the first pieces of work revealing there was little difference between teams that “established” the run early in games and those that didn’t. It also showed most teams with high run totals weren’t running early, rather running late in games after they took the lead. This refutes the type of analysis that says “TEAM X is 8-2 when they run the ball 25 or more times” or something similar with a specific running back. The truth is Team X or Running Back X typically only get to 25 carries if there is already a lead in place.
Last year, the average pas-run ratio across the league was 59-41. In the first half of games when teams are trying to set the tone for the game, it was 60-40. In the fourth quarter with a lead, teams shifted to 34-66.
It’s not just the number of carries for a star running that is misconstrued, but even the value of that back is not as high as once thought. When predicting the success of a running play, we’ve learned the specific running back is one of the least important aspects. One of the reasons for this is not that running backs aren’t talented players. It’s exactly the opposite. There are so many talented players at the position that there’s arguably a smaller gap between the top of the position and average at running back than any position on the field.
Few running backs have the ability to overcome deficiencies in other areas and while you think of the “few,” it’s probably even a smaller number than you think. We know the offensive line matters and we now know the situation in which a back is asked to run matters, too.
Studies have shown that the number of defenders in a box can predict over 80% of a running back’s yards per carry. The same studies have shown how a team’s offensive personnel is the biggest factor in how a defense decides to stack the box. For example, a defense is more likely to put more defenders in the box when the offense comes out in 12 personnel (one running back, two tight ends, two wide receivers) or 21 personnel (two backs, two tight ends, two wide receivers) than it would against 11 personnel (three receivers) or 10 personnel (four wide receivers).
Those heavier personnel packages make the play look like a run, so the defense is more likely to line up to stop it. Think about how many times a team lines up in a tight heavy formation on a 3rd and 2 and runs into a brick wall because of how many defenders are ready to stop the run attempt. Now, think about the early success Sean McVay had running the ball with a spread out offense or how good the Cardinals’ run game was this past season under Kliff Kingsbury.
Teams can give themselves an easier time running the ball by spreading out the defense to open up the box. The biggest advantage goes to teams that can run well from passing looks and pass well from run looks. All of this is a big reason why you’re going to read Warren Sharp stress personnel frequencies and tendencies so often in the upcoming team chapters.
Maybe most importantly we’ve learned how little the success of a run game has to do with the success of play-action. Play-action is an instant upgrade for a passing offense. Last season, passing attempts without play-action averaged 6.79 yards per attempt with a 4.2% touchdown rate and 2.5% interception rate. Play-action passes averaged 8.5 yards per attempt with a 5.3% touchdown rate and 1.8% interception rate.
Research has shown that the number of rushing attempts or the success of a run game had little to do with play-action success.3 The easiest way to be good at play-action is to use play-action well and much of that involves making the pass look like a run. When you hear coaches like McVay or Kyle Shanahan say how everything is built off the run game, they’re talking about the look. Those play-action passes need to look exactly like the runs, regardless of whether those runs have been successful or not. Player tracking data also has shown that linebackers react similarly to play action regardless of when it is called. It’s another idea that inherently makes sense. Linebackers are taught to read keys from the moment they learn the position. As long as the run fake is well-executed, it’s likely to have the intended result.
Of course, this isn’t to say there’s no value in the run game. There’s value to be had, but it’s less on early downs and early in games like the old school mentality would suggest. Two places where runs are more valuable are on third and fourth and short and in the red zone.4
New stats to replace your old ones
With so many new metrics coming out, it can be overwhelming to keep track. But there are some simple ways you can upgrade your numbers.
Throughout this book, we will walk through more advanced metrics. You can find definitions of many of those metrics on the page entitled “Team Chapter Layout and Definitions” before the team chapters begin.
But let’s walk through a number of guiding principles you should take with you as you study the NFL during the 2020 season and beyond.
- Stop Using Raw Totals
Whenever you hear a TV announcer says a team ranks 12th in offense, they’re talking about raw yardage totals 99% of the time. Rarely is that a great indicator of team performance, especially if it’s used in the middle of a season when teams haven’t played the same number of
games. Even per game numbers can be somewhat misleading. Yards and points do go together, but empty yards at the end of games can help a team’s ranking without doing much to help the team win or be a good offense. Instead, you can use per play or per drive metrics. Those give a better indicator of both production and efficiency on each side of the ball.
For example, the Tampa Bay Buccaneers were third in total yards on offense in 2019, but they were nowhere near the third-best offense in the league. They were closer to average (15th) in yards per drive. The Buccaneers just had a lot of drives because of the turnovers created by the offense and short fields given to the defense. There was a lot of late-game passing in Tampa Bay games last season.
This is the easiest thing to change and the best first step for a widespread understanding of smarter numbers.
(Everything here can also be said for time of possession)
- Use Completion Percentage Over Expected (CPOE) rather than Completion Percentage
Over the past few seasons, completion percentages have risen each year. Before 2015, no quarterback completed over 70% of his passes. In 2019, three did. The rise in completions has coincided with a rise in shorter passes. All that has impacted how we should look at raw completion percentage.
Take Quarterback A who completed 16-of-25 attempts but threw his average pass 6.5 yards beyond the line of scrimmage to wide open receivers. Then take Quarterback B who completed 13-of-25 passes but his average throw traveled 14.5 yards past the line of scrimmage into tightly covered receivers. Which quarterback had the more impressive performance?
Those numbers were real Week 12 statlines from Jacoby Brissett (Quarterback A) and Russell Wilson (Quarterback B). NFL Next Gen Stats uses player tracking to monitor things like depth of target, time to throw, and receiver separation to create a CPOE metric. On this example, Brissett completed 64% of his passes but was expected to complete 72% given the situation (- 8%). Meanwhile, Wilson only completed 52% of his passes but was expected to complete just 49.9% (+2.1%).
CPOE is easily available on the Next Gen Stats site and is also available in multiple other places. There will be CPOE graphs included in the team chapters in this book.
- Don’t be afraid of QBR
When ESPN came out with QBR as an alternative to passer rating, its effectiveness was questioned. But through the years, it has proven to be one of the better publicly available single- number metrics for quarterback performance.
The best way to view QBR for single games is as a de facto win probability metric and it’s much easier to understand that way. The 1-100 scale shows the percentage of games a team is expected to win with the quarterback’s performance. A 99.8 QBR doesn’t necessarily represent one of the best quarterback games ever played, but rather that a team with that type of play at the position would be expected to win 99.8% of the time — there’s a huge difference and the latter is significantly more useful.
QBR can overweight rushing on a seasonal level, but the metric will still give one of the better looks we have at quarterback play.
- Focus on QB hits instead of sacks
Sacks have long been the standard for pass rushers. But they happen so infrequently, we’re usually judging full seasons of pass rushing on just a handful of plays. Pressure rates are the best way to figure out a pass rusher’s production but the problem is pressure isn’t an official stat and can range from outlet to outlet. But for a better boxscore stat, take a look at quarterback hits.
Getting to the quarterback is a skill but taking him down for a sack takes a lot more luck than just being in the right place. A quarterback who gets the ball out quickly can run a low sack rate because he’s not giving the defense an opportunity to get a sack (this is also why sack rates should be considered more of a quarterback stat than one for the offensive line).
Quarterback hits are still counted when the defender gets to the quarterback but the ball comes out. A hit is good process of doing what needed to be done to get to the quarterback regardless of whether or not a sack was recorded. A high number of hits can also suggest sacks are coming soon. I’ve previously done work on how sacks per quarterback hit can fluctuate from year-to-year and how a player with a high hit total and low sack numbers is a good bet to increase those the next year.
Embrace Imperfection Using Variety and Context
There are no perfect stats. As mentioned in the EPA section above, we don’t have one number that can succinctly encapsulate everything happening on the field. But also, that fact should not cancel out all of the progress that has been made to better reflect what does happen each Sunday, and Monday, and Thursday, and sometimes Saturday.
As much as possible, you should use a variety of statistics to evaluate performance. This is why, for example, when analyzing each player’s performance by personnel grouping in the team chapters, Warren shares three metrics: EPA/play, success rate, and yards/play. All three in concert will provide a far clearer picture as to the performance this player delivered than any of these metrics would deliver individually.
Our most powerful tool in football analysis is knowing what we don’t know. Football is a small sample size sport, which is why context must be applied to all metrics in order to determine if the relevant conclusion to be drawn could be valid. It’s almost as much an art as it is a science.
Keep Getting Smarter
There are a number of other metrics we could have explored (and I encourage you to do so). The game of football is consistently evolving and so is the analysis that surrounds it. The game doesn’t look like it did in 1997, so we should stop talking about it the same way we once did. We’re getting smarter about how we talk about football and the best thing about that is you don’t even have to be that smart to understand it. Trust me, take it from a Communications major.